Today, AI has become one of the most important topics for companies, which further increases the competition in the market. Very high budgets are poured into AI and analytics projects by companies each year, and these budgets are expected to grow in near future.(1) Despite the high budgets spent, 87% of data science projects do not even make it into production, according to the report published by VentureBeat.(2) According to Gartner, only 20% of analytical models produce business outcomes.(3)
Various changes over time, explained below, are among the most important reasons why AI projects have such a low success rate despite the excess of resources spent.
• Concept Drift (reduced ability of existing features to explain the predicted value) • Data Drift (change in distribution of existing features) • Algorithm Drift (assumptions becoming inadequate, change in business needs)
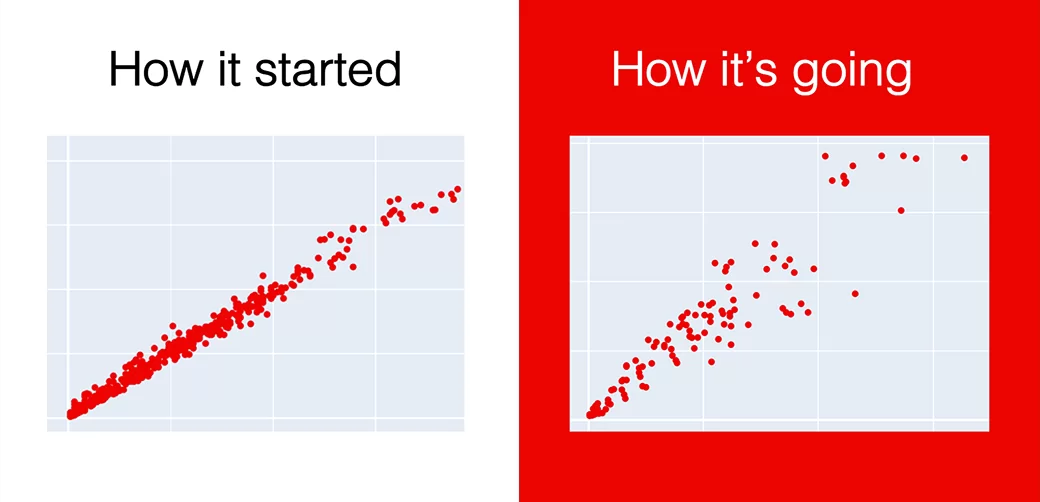
Concept Drift
The decrease in the explainability of the target variable, which is tried to be predicted or classified in analytical models, with the existing features is called concept drift.
Many reasons such as semantic or unit change in the target variable can lead to concept drift. Let’s say we are working on a fraud detection project. Suppose that while training the model, the target variable describing the fraud transaction creates an input as 1. After the model is integrated, if data is collected as 1 represents non-fraud transactions, the output of the model will be distorted. As an example of unit drift, in the process of integrating the target variable in grams, which was in kilograms during model development process, will suddenly increase the target variable’s value 1,000 times, which will seriously affect the model’s performance.
It is critical to monitor the models and be proactive to avoid these drifts. It is necessary to be investigative in order to be aware of the drifts and evaluate them. It should be determined whether these drifts are one-time or continuous events. Once the diagnosis is made, it may be necessary to modify the relevant target variables and retrain the model.
Data Drift
One of the most important reasons for the degradation of model performance is data drift. Machine learning models use the features in the dataset to make predictions. A data drift in this dataset can significantly affect model’s performance. Since this effect will be cumulative, it will cause deterioration in all model outputs afterwards. For this reason, just like concept drift problems, regular monitoring of data drift and intervention if necessary, will ensure the continuity of model performance and produce healthy results.
Some examples of potential data drift cases are as follows: • The emergence of unusual conditions such as Pandemic etc. • Corruption of the tool (sensor etc.) that collects the data • Seasonality effects • Change of data structure rules
Apart from these situations, data drift may occur due to different reasons as well.
Algorithm Drift
Artificial intelligence models are designed in line with business needs and assumptions at the time of development. For this reason, it may be insufficient in responding to new needs that may occur over time or to changing ways of doing business. In order to prevent this situation, business needs should be analyzed regularly, and the competence of the model should be tested in this context, and the algorithm should be adjusted if necessary.
Why Continuous AI is a Must?
Completing the development process of an artificial intelligence model or analytical project does not guarantee that the benefit will be consistently high. For the spent budget to reflect more positively on business outcomes and for the developed models to be more efficient and useful, the relevant models should be constantly monitored and analyzed by expert teams. In this way, the development needs can be determined and the benefit from the model can always be kept at the highest level.
Sources: (1) https://www.forbes.com/sites/davidjeans/2020/10/20/bcg-mit-report-shows-companies-will-spend-50-billion-on-artificial-intelligence-with-few-results/ (2) https://venturebeat.com/2019/07/19/why-do-87-of-data-science-projects-never-make-it-into-production/ (3) https://blogs.gartner.com/andrew_white/2019/01/03/our-top-data-and-analytics-predicts-for-2019/